
The second possible cause is that the USB connection is not established.
#Principal data feed instacal software
To fix this problem, download the latest revision of software from this website. Measurements will thus not be maximally accurate. If you continue to operate the software, default gain parameters will be used in place of the correct ones. The first possible cause is that the MouseOx control unit was manufactured after the date of the software release and the calibration values are not available in the software look up table. The Calibration Not Confirmed error message will occur for one of two possible reasons. MouseOx® is running fine and then it just stops working.First time trying to run MouseOx® and software will not run.Calibration not confirmed error message.We have listed the most common issues and linked to the solutions below. $$\overline_j$, is the eigenvector corresponding to $\lambda_j$.The MouseOx Plus® is easy to use but there are occasions when customers have difficulty. To fix notation, let measurements $x_1, \dots, x_n$ be taken on $M$ samples $s_1, \dots, s_m$.įor each $x_i$ we calculate the average, or mean To be specific, suppose the data set consists of $n$ measurements taken on $M$ samples geometrically it would be a set of $M$ points distributed in $n$-dimensional space, where each sample is a point and its $n$ measurements are its $n$ coordinates. ( Astronomers describe the general procedure as “finding the principal axes of inertia of the cloud of samples about their mean in feature space.”) The a priori unlikely combination of basic elements of statistics, multivariable calculus and linear algebra that makes it work will be outlined in this section. In the “Earliest … burial” example, PCA gives a useful 2-dimensional representation of a cloud of points in a 13-dimensional space. To visuaize their ensemble of measurements (80 data points, each with 13 components), “e performed PCA of EDXRF concentrations for the thirteen major, minor, and trace elements more frequently detected (Si, K, Ca, Ti, Mn, Fe, Zn, Ga, As, Rb, Y, Zr and Ba).” As they report, “The burial pit samples markedly differ from layer 19.” The basic mathematics “The burial fill is a mix of ferruginous silt and sand, compositionally similar to the top of layer 18 and the base of layer 17, and different from layer 19, in which the pit was excavated.” They confirmed the difference by measuring the concentrations of 13 chemical elements occurring in the burial fill and in those three layers. that a pit had been dug to receive the child’s corpse. In documenting the 78,000-year-old grave of a child in Panga ya Saidi, Kenya, they needed to establish that there had actually been a burial they did this in part by showing that the earth surrounding the skeleton was different from the surrounding earth at that level (layer 19) in the archaeological excavation, i.e. The authors are an international team of 36 led by María Martinón-Torres, Nicole Boivin and Michael Petraglia. Image adapted from Nature, 593, 95-100, Extended Data Figure 4Įarliest known human burial in Africa was published in Nature on May 5, 2021.
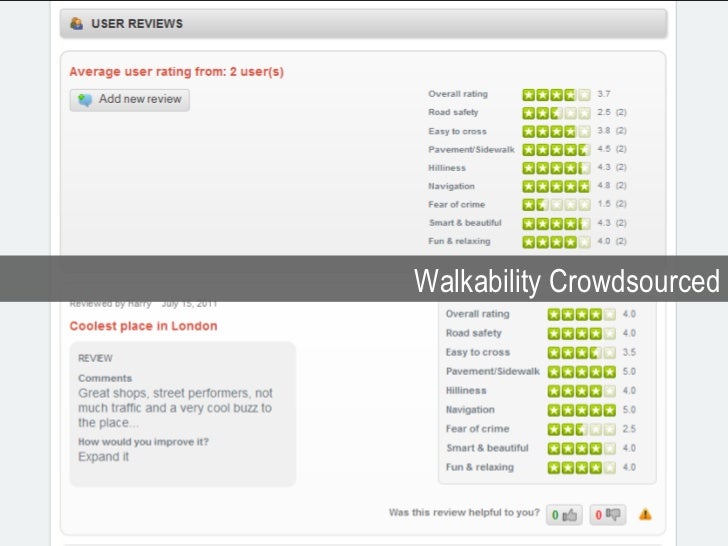
The projection shows a clear distinction (highlighted by the superimposed 95% confidence ellipses) between samples from the burial pit (red dots) and samples (purple dots) from outside the pit at the same level (Layer 19) of the excavation. Distinguishing nearby soil samples in a 78,000-year-old burial In this PCA, 13-dimensional data from some 80 soil samples are projected into the plane spanned by their two principal components. In this column we will look at PCA in three recently published applications (to anthropology and paleontology), and go through the mathematics that makes it work. One can construct artificial examples where all the dimensions must be considered, but very often, especially in applications to the life sciences, useful low-dimensional projections exist and allow humans to grasp a data set that would otherwise be inscrutable.
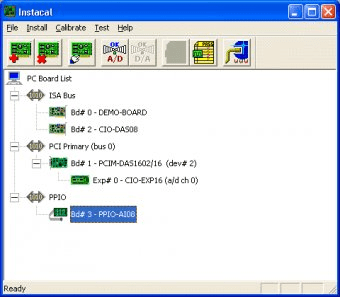
The goal of PCA is to produce the most useful possible 2 or 3-dimensional projection of a high-dimensional data set-most useful in that the smallest amount of information is lost by the projection. Human minds are good at recognizing patterns in two dimensions and to some extent in three, but are essentially useless in higher dimensions. Principal component analysis (PCA), an algorithm for helping us understand large-dimensional data sets, has become very useful in science (for example, a search in Nature for the year 2020 picks it up in 124 different articles). Very often, especially in applications to the life sciences, useful low-dimensional projections exist and allow humans to grasp a data set that would otherwise be inscrutable. Principal Component Analysis: Three Examples and some Theory
